Over the last decade, large international efforts have revolutionized our understanding of how our genome functions in normal and disease states.
The Computational Biology Team focusses on utilizing publicly available large datasets in combination with innovative computer algorithms to 1) improve diagnostic rates in children suffering from undiagnosed diseases and 2) discover new disease mechanisms and biomarkers in childhood cancers.
Our aim is to understand how individual bases in our genome predispose, alter and interact in normal and disease contexts.
We focus on analyzing large public omics datasets using machine learning algorithms to understand dynamics of genome activity – which sequences are used when and where. Linking this data back to the genetic information of a patient is allowing us to discover the molecular causes of diseases and is key in predicting future disease and treatment outcomes.
Team Highlights - Rare diseases
- Anderson, Denise, and Timo Lassmann. "An expanded phenotype centric benchmark of variant prioritisation tools." Human Mutation 43.5 (2022): 539-546.
- Lassmann, Timo, et al. "A flexible computational pipeline for research analyses of unsolved clinical exome cases." NPJ genomic medicine 5.1 (2020): 1-11.
- Anderson, D., Baynam, G., Blackwell, J. M., & Lassmann, T. (2019). "Personalised analytics for rare disease diagnostics". Nature communications, 10(1), 1-8.
- Boycott KM, Hartley T, Biesecker LG, Gibbs RA, Innes AM, Riess O, Belmont J, Dunwoodie SL, Jojic N, Lassmann T, Mackay D. "A diagnosis for all rare genetic diseases: the horizon and the next frontiers". Cell. 2019 Mar 21;177(1):32-7.
- Anderson, Denise, and Timo Lassmann. "A phenotype centric benchmark of variant prioritisation tools." NPJ genomic medicine 3.1 (2018): 1-9.
Team Highlights - Functional genomics
- Hon, Chung-Chau, et al. "An atlas of human long non-coding RNAs with accurate 5′ ends." Nature 543.7644 (2017): 199.
- Arner, Erik, et al. "Transcribed enhancers lead waves of coordinated transcription in transitioning mammalian cells." Science 347.6225 (2015): 1010-1014.
- Forrest, Alistair RR, et al. "A promoter-level mammalian expression atlas." Nature 507.7493 (2014): 462.
- Andersson, Robin, et al. "An atlas of active enhancers across human cell types and tissues." Nature 507.7493 (2014): 455.
- ENCODE Project Consortium. "An integrated encyclopedia of DNA elements in the human genome." Nature 489.7414 (2012): 57.
- Djebali, Sarah, et al. "Landscape of transcription in human cells." Nature 489.7414 (2012): 101.
- Maida, Yoshiko, et al. "An RNA-dependent RNA polymerase formed by TERT and the RMRP RNA." Nature 461.7261 (2009): 230.
Featured research
Published in Science
Published in Nature
Team leader
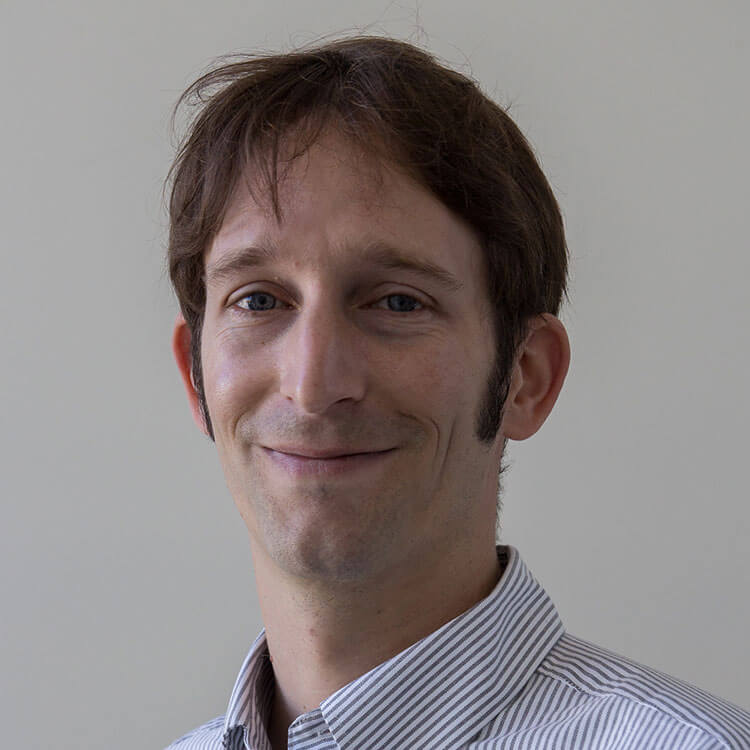
BSc (Hons) MSc PhD
Feilman Fellow; Program Head, Precision Health and Head, Computational Biology
Team members (3)
Chin Wee
PhD student
Timothy Chapman
Research Assistant
Kathryn Farley
PhD Candidate
Computational Biology projects
Featured projects
Centre for Advanced Cancer Genomics (CACG)
Current technologies to understand which genes are turned on or off only work on large amounts of biological samples. As a consequence all measurements we receive represent averages across multiple cell types present in the sample. The situation is comparable to studying the contents of a bowl of fr
Undiagnosed Diseases Program (UDP) and Bringing the benefits of precision medicine to children in Western Australia
We have started a project utilising whole genome sequencing of undiagnosed children living in WA to provide a definitive diagnosis. A major challenge here is that the role and functions of the inter-genic regions of our genome (the remaining 98%) are relatively poorly understood.
Other projects
An unbiased exploration of the human regulatory landscape